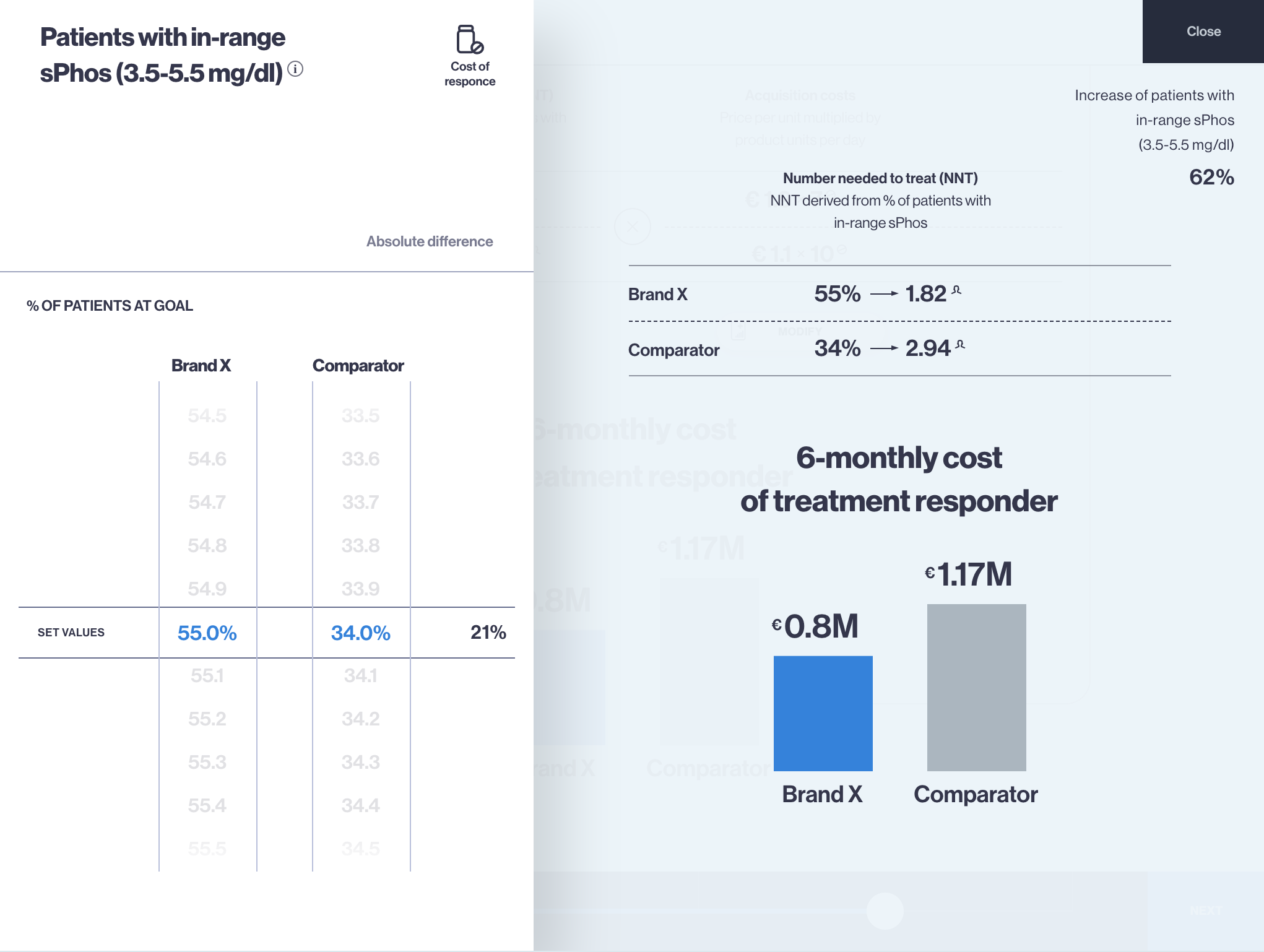
This article is inspired by the exciting idea of data interoperability in health economics modelling process, which means sourcing (fetching) inputs for models in real-time, using modern software infrastructure capabilities. On a larger scale, development of naming conventions for health economic model inputs would be required, so this is also a call for action for researchers working in the health economics modelling domain.
Interoperability – the ability of computer systems or software to exchange and make use of information.
Most of the modellers and consultants working in the field of Health Technology Assessment (HTA) and economic evaluations of healthcare technologies are familiar with the labour intensive process of manual updates/localisation in cost-effectiveness or a budget impact model. In particular, the treatment effect data needs to be validated and approved by multiple parties before it is published and used as a model input to project future health and cost outcomes beyond the lifespan of a clinical study.
What are the true costs of a manual model update process?
In a way, we want to question and challenge the delays related to manual updates of model inputs and current way of operationalising the data in health economic models.
- How much time is spent on average to update the model ?
- What are the hidden and true costs of not making a timely resource reallocation decision due to delays in model update?
- Should all data be manually reviewed before publishing or updating the model results?
- Which data can currently be sourced without a human reviewer and which data would require a similar scrutiny after more rigorous standardisation process?
HTA bodies might require scheduled updates to reevaluate drug price and value based on the new published evidence. Now, imagine if in the era of information technologies, connectivity and Application Programming Interfaces (API) becoming a standard, cost-effectiveness, budget impact, return-on-investment and portfolio optimisation models can become self-updatable! I’d say – that would be wonderful, but hard to imagine, as it seems almost impossible to agree on several aspects, even within a single country setting:
- Data classification standard and naming convention standard for input parameters
- Data sources and their level of acceptance and relevance for a decision problem
- The everlasting need for human interpretation of complex healthcare data and heterogenous results of clinical trials.
The question is would all these and especially the last point (heterogeneity and complexity of interpretation) apply to all types of health economic model inputs? To answer this question, let’s try to go through a simple classification of key data inputs for health economic models and try to estimate the degree of necessity for an additional human review and interpretation (Figure 1).
Figure 1. Types of health economic model inputs and their applicability for real-time fetching.
At first sight it seems that some of the health economic model data could be inputted in the model without a human interpretation. This could specifically be true true for:
- Disease incidence and prevalence. Can be sourced from nation-wide sources (when available) using ICD codes classification
- DRG costs/tariff. Are updated and published regularly. Can be sourced, once the new update is available
- Drug prices and market shares: Usually health economic models are populated with list prices, so why not to fetch them from national country-specific published price lists or other trusted source together with utilisation data to derive market shares (uptake rates of drugs) automatically?
- Normative utility values. These are standard values and they do not changed very frequently. They can definitely be sourced automatically
- Number of eligible patients. Can be location specific and sourced from a local EMR for a particular indication related to the drug
Now the hard ones, they could definitely require a more a more in-depth human review and interpretation. However a dedicated system of alerts could flag that the new data became available and better resource allocation decisions could be made (i.e. significant change of order in the cost effectiveness league table).
- Efficacy data:
- From RCT – Risk ratios for primary and secondary endpoints can be sourced from clintrials.gov on the fly (if available)
- From real-world setting – linkage of the economic model to a Phase IV study or registry could provide a real-time dynamic view on the cost-effective price (given a particular cost-effectiveness threshold). Data rich registries can also be useful in determine the amount of resources used (healthcare use, appointments, medication, surgery…) that mimics real world use as they would be collected in the entire (or representative) population of patients receiving treatment (more naturalistic) unlike RCTs that have limited and selected samples and often generalisability issues.
- The need to update meta-analysis – this is a difficult task involving the review of a wider body of evidence and checking for risk of bias, heterogeneity, consistency, internal validity and other quality of evidence and certainty criteria. On the other hand, it could be most feasible to update meta-analyses automatically, as standard nomenclature, reporting and a set of validation rules exist (i.e. GRADE). On of the big advantages of automation in this area is to have a living meta-analysis/network meta-analysis, which is being properly validated and constructed from the beginning. It could be updated as new evidences comes in review decision space.
- Utility values and quality of life data. There is no single source for utility data, so it needs to be sourced from studies identified in the literature. Can the literature reviews be automated? Maybe to some extent. Can a centralised registry of all utility weights and a duration of episode be standardised and become available for global use in health economic models?
Modellers (regardless if they are in the industry, consultancy or academia) must ensure that the data originates from the most valid sources. In some cases, they may need to re-conduct a meta-analysis to add another source of efficacy/effectiveness data in the “forest plot”. In such a case, the concept of “on-the-fly updates” becomes even harder to imagine. Unique studies could be automatically obtained upon status change to” Completed” at clintrials.gov. Does that, together with the development of unified model inputs naming convention, sound like a first step in such a direction?
How the new process could look like?
Of course, the part of changes to the model data must be reviewed and approved by system administrator in a way similar to track changes functionality with “Reject” and “Approve” options for complete audit trails and logging purpose.
Proposed examples for coding for the development of naming conventions:
- Prevalance_DiseaseICD10Code_AgeGroup
- Utility_DiseaseICD10Code_AcuteEpisode_AgeGroup
- HospitalisationCost_DRGCode
- DrugCost_Year_Country
Input parameters in health economic models are already coded in a similar way but there is no formally agreed standardisation. This poses the question is – are we, as global health economics leaders ready for such unification and standardisation? We’d appreciate to see an ISPOR Task Force on such an interesting, challenging, but definitely a much needed topic. Health economic models are criticised for their lack of transparency and high level of complexity, so why don’t we make this step to improve these perceptions?
Development of unified classification and naming conventions are at the centre of effective healthcare management and regulations. A good example is the FDA preferred substance names and their identifiers. The FDA now argues that the eCTD content does not follow the development flow, contains unstructured data, and varies in the level of granularity provided. The pdf format has its own limitation in terms of data mining, making lifecycle management challenging. This is understandably an issue that has been raised and acknowledged by the industry. Suggestions for alternate formats and platforms have also been made.The draft document circulated by FDA provides a set of key data elements and terminologies associated with PQ/CMC subject areas and uses Global Substance Registration System (G-SRS), Data Universal Numbering System (DUNS), Structured Product Labeling (SPL), Unified Code for Units of Measure (UCUM) and other data fields are to be coded based on HL7. Similar data standardisation efforts were also taken by HDR UK.
Final thoughts
It is hard to underestimate the benefits of interoperable health economic models. They lead to timely decisions, better access to much needed treatments and health gains arising from effective decisions that are made faster by simplifying and automating the evidence update process. The current time lag between data availability and model publication is at least 6-12 months. It takes time to review all model data, approve it with internal and external stakeholders, following the strict compliance process at all levels (CRO, Consulting, Manufacturer and finally the assessing HTA bodies). Can the time lag be shortened approximately by half, by providing half of the data in automatic interoperable format? This is a rhetorical question from our humble health economics IT standpoint.
It is also true that when a model is prepared and published in a peer-review journal, it’s results remain static and valid only for a certain, very limited amount of time and a particular setting (country). Of course publications provide a great way of sharing a scientific knowledge, but true to say don’t easily allow to reproduce modelling studies, as the source code is often not published or the model structure is impossible to reproduce.
We should use automation to facilitate human judgment, and to free-up time for humans to do what only humans can do.
Most of the benefits arising from interoperability lie within the provision of early access for patients and time savings related to unnecessary actions for requesting new data and manual copy-paste and updates. The data infrastructure can be setup in a way that Excel or web-based health economics models source data on the fly from a centralised database location where all the data is classified and made available and ready for exchange with health economics models. By linking registries and other validated sources of real-world data, decision-makers can obtain insights on a cost-effective drug price much earlier and make much better and timely resource allocation decisions.
Interested to learn more? Contact us to explore how we can make models self-updatable within your organisation.
Author: Oleksandr Topachevskyi;
Ackownlgedgment: Many thanks to Carina Schey, Rui Martins, Joan Mendivil and Jari Kempers for their valuable inputs and editorial comments!