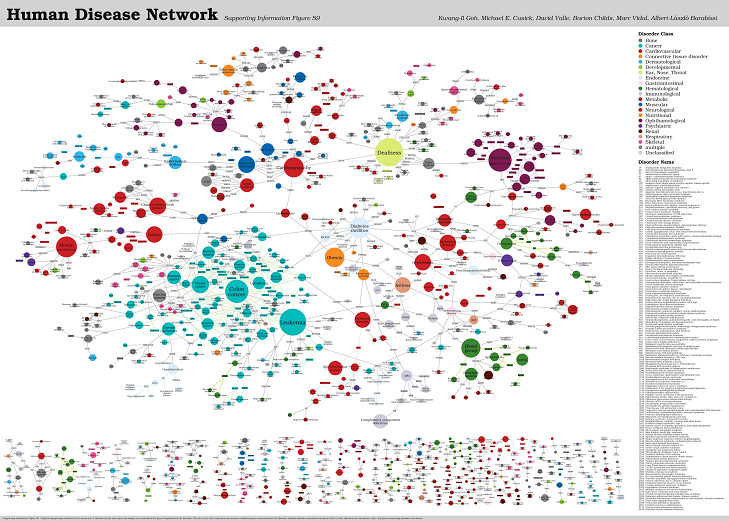
The ‘big data’ matter has lately been widely discussed within the various businesses and industries, such as IT, chemical, pharmaceutical, metallurgical, financial, oil and gas, consumer, high technology, retail etc. No wonder it even managed to create a significant hype, the promises of big data acquisitions are very tempting, but the failure rate is high as well.
We tried to analyze different researches regarding ‘big data’ and form our own opinion on the subject.
“Big Data is like teenage sex: everyone talks about it, nobody really knows how to do it, everyone thinks everyone else is doing it, so everyone claims they are doing it”
Let’s start with the definition of ‘big data’. ‘Big data’ is a term used for data sets so large or complex that traditional data processing software is unable to capture, curate, manage, and process data within a tolerable elapsed time. Challenges also include analysis, search, sharing, storage, transfer, visualization, and information privacy. The term often refers simply to the use of predictive analytics or other certain advanced methods to extract value from data, and seldom to a particular size of data set.
Challenges or common mistakes in the area of big data usage, analytics and decision-making:
Asking wrong questions. Data science is very complex and requires various sector specific skills and knowledge. Technology alone won’t solve the problem. In fact, most failed data analysis efforts derive from asking the wrong questions and using wrong data.
- Lacking the right skills. Many big data projects fail because of the incompetency or lack of corresponding knowledge of the executors.
- Problem avoidance. This includes data projects requiring managers to take action that they don’t really want to do, like the pharmaceutical industry not running sentiment analysis because it wants to avoid the subsequent legal obligation to report adverse side effects to the U.S. Food and Drug Administration.
- Management inertia. Many managers claim that while making decisions they trust their industry knowledge and even intuition more, than computers and calculations.
- Wrong usage. This includes both using traditional technologies for big data tasks and starting big data projects which the companies are not yet ready to execute.
- Unanticipated problems beyond big data technology. Big data projects have many components, like human factor, storage, sharing, transfer, capturing etc. and one of them failing means failure of the whole project.
- Big data silos. Big data vendors often talk about “oceans” and “lakes” of data, while they look more like “puddles” which are yet to be united into something bigger. Lack of data sharing often takes place even within a single company between its departments.
Solutions and suggestions to avoid getting in the big data failure:
- Clearly understand data needs and requirements. Focus on a few measurable and robust data (business outcome measures, indicators,) rather than on ocean of available data.
- Do not hire, but train analytical team internally, within own company environment. Those are usually called data scientists. Human factor is the key thing that makes all the technology work. Training own data specialists internally makes more sense, than hiring data scientists externally, as learning to use data software is easier than learning the whole business
- Find and prove causal relationship between X and Y. Identify causal relationship between input factors to change (labor, technology) in business processes in order to influence outputs (profits, units sold and other KPI metrics).
- Develop internal machine learning algorithms. It means creating algorithms which self-learn from the data they get and self-develop according to the knowledge they acquire. Machine learning principle is often considered as the basis for Artificial Intelligence.
- Finding statistically meaningful data. Making sure the sample is big enough and representative of subject of interest.
- Don’t store information, share it internally. McKinsey analysts suggest that information storage often slows organisation development, while information sharing can encourage company’s employees, clients or partners to give useful advices and make more accurate assessments.
The proliferation of so-called ‘big data’ and the increasing capability and reducing cost of technology are very seductive for retail financial services organisations seeking to improve their customer engagement and operational performance. But many simply do not appreciate the real costs – in terms of money and time – that burden ‘big’ approaches to big data programmes. And very few understand that the strength and quality of customer engagement bear little relation to the tools that have been bought. Rather than rushing into big data programmes, organisations need to invest in a ‘lean’ approach to data and analytics, which will align all business capabilities, including strategy, people, processes and technology, towards a more socially connected customer.
Big data. Time for a lean approach in financial services” by Deloitte