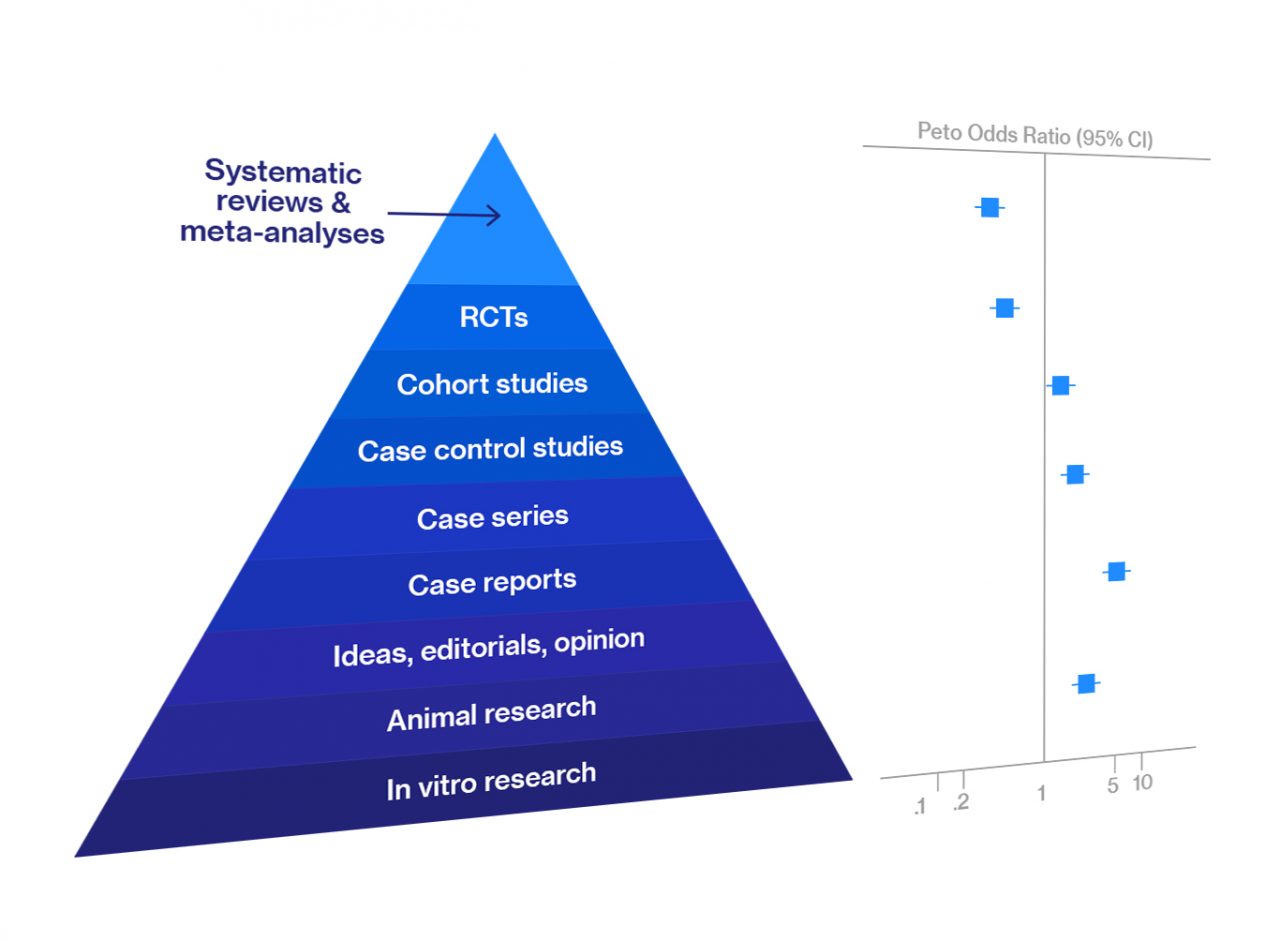
Meta-analysis and systematic review are at the top of the hierarchy of evidence for data reliability in various types of biomedical research. They are an integral part of evidence-based medicine. Most clinicians begin searching for the best, evidence-based evidence to make informed decisions by visiting the Cochrane Library, which is regularly updated with more and more meta-analyses and systematic reviews. Why meta-analysis data are so important is discussed in this article.
What is meta-analysis
Meta-analysis is a scientific research methodology consisting of the analysis and synthesis (combination) of data obtained from previously conducted independently from each other studies on the same problem. Most often, it is used to assess the clinical effectiveness of therapeutic interventions. For this purpose, the results of two or more randomised controlled trials are combined.
A meta-analysis uses statistical methods to obtain an overall estimate of the effect. In doing so, a qualitative meta-analysis involves:
- Examining all studies on the problem in question eliminates the influence of studies and opinions that are more popular at a given time.
- Assessing the heterogeneity (heterogeneity) of the meta-analysis. When applied to meta-analyses, heterogeneity refers to differences between studies, which may be due to the use of different methods of statistical data processing (statistical heterogeneity) or to differences in key characteristics of participants, interventions used, clinical outcomes, or criteria for their evaluation (clinical heterogeneity). These types of heterogeneity may make it impossible to generalise the data in a meta-analysis.
- Determination of the informativeness of the main results by sensitivity analysis.
The importance of meta-analysis in evidence-based medicine
Multiple research groups in different parts of the world study many medical issues simultaneously. And often, the results of these studies are contradictory, complicating clinical decision-making. This understanding led to the development of evidence-based medicine, an approach to medical practice in which decisions about any medical intervention are made based on available evidence of effectiveness and safety, with such evidence being evaluated, compared, summarised, and widely disseminated for the benefit of patients.
The main feature of evidence-based medicine is a critical appraisal of the available evidence. Meta-analysis allows the results of several independent studies to be combined and assessed unbiasedly. Therefore, this analysis occupies an important place in evidence-based medicine.
Its advantages include:
- the ability to consolidate the results of multiple studies on one particular topic, even if their results contradict each other;
- no need to re-collect data;
- indicates that the sample is more diverse than expected based on sample diversity;
- controls for diversity between studies;
- can explain inconsistencies between data;
- allows for increased statistical power of studies;
- works in an environment of excess information – a large number of articles are published each year;
- summarises multiple studies and is, therefore, less dependent on individual findings than individual studies;
- can detect systematic errors.
Figure 1. Quality of evidence ranking
Because of this, since 1979, when Canadian scientists began to evaluate the effectiveness of an intervention according to the quality of the evidence obtained, data from meta-analyses and data from systematic reviews have been at the top of the hierarchy of evidence.
In addition to synthesising all currently available information and bringing new insights into the most effective treatments or diagnostics, meta-analysis data sets the vector for new research. This is because the evaluation and recommendations that follow a meta-analysis largely depend on the quality of the studies conducted previously. If they were conducted superficially, biassed, or poorly implemented, the researchers who conducted the meta-analysis would conclude that the evidence of effectiveness needs to be stronger and new, better studies are needed.
Systematic review VS Meta-analysis
Systematic review and meta-analysis are related, and both involve the study of data. Therefore, these concepts often need clarification. In fact, they are different types of work with different objectives and methodologies.
Systematic review is a type of scientific work which consists of the study of all scientific research on a certain topic at the time of the analysis. The study’s main purpose is to summarise, analyse and evaluate the information obtained, and get an answer to the specific question that caused the review.
Meta-analysis is a method of statistical analysis which allows you to quantitatively combine and evaluate the results of different but similar studies. The main purpose of meta-analysis is to integrate data from different independent studies, group them together, and determine a general trend. Meta-analysis can either be a stand-alone study or used as one method to quantitatively synthesise the results of individual scientific papers within a systematic review.
The difference between a systematic review and meta-analysis is that systematic review is the first step that includes development of search strategy and protocol, whilst meta-analysis is a statistical technique that aggregates studies that pass inclusion criteria and calculates an averaged estimate and respective confidence intervals.
The process and methods of meta-analysis
Many guides, manuals, and recommendations for conducting systematic reviews and meta-analyses have already been developed that vary slightly in their approach, but each of them includes 5 common steps.
- Task Formulation. This step defines the clinical research question and hypothesis. The likely clinical relevance is explained, and the study design and analytic plan are justified.
- Development of criteria for inclusion/exclusion of original studies in the meta-analysis:
- inclusion based on qualitative criteria, e.g., presence of randomization and blinded controls in clinical trials;
- selection of individual studies by subject, e.g., treatment of breast cancer;
- inclusion or noninclusion of unpublished data.
- Decisions about which dependents are not included in meta-analyses:
- differences (discrete data);
- averages (continuous data).
- Systematic search and selection of original studies according to previously defined inclusion/exclusion criteria.
- Effect evaluation.
The key task in the last step is to select and apply a suitable method to create a numerical model that will allow a comparison of effect sizes in different studies. The choice of analysis method is determined by the type of data being analysed (binary or continuous) and the model type (fixed effects, random effects).
Binary data are usually analysed by calculating matched samples’ odds ratios, relative risk, or risk differences. All of these measures characterise the effect of the interventions.
Continuous data are usually the ranges of values of the studied characteristics or the unstandardized difference of weighted averages in comparison groups if outcomes were assessed in the same way in all studies. However, if outcomes were assessed differently (e.g., using different scales), the standardised difference in the means of the comparison groups is used.
Popular methods and approaches for meta-analysis are:
- Mantel-Hansel method
- Random-effects model
- Bayesian meta-analysis
- Cumulative meta-analysis
- Regression meta-analysis
- Generalisation of estimates of the informativeness of diagnostic methods obtained in different studies
After obtaining a generalised estimate of the effect size, it is necessary to determine its stability. For this purpose, sensitivity analysis is performed. Depending on the specific situation, it can be performed based on several different methods, for example:
- inclusion and exclusion of studies performed at a low methodological level from the meta-analysis;
- changing the parameters of the data selected from each study under analysis;
- excluding the largest studies from the meta-analysis.
There are also ways to assess the completeness of the identification of studies included in the meta-analysis. Usually, incomplete detection is associated with the occurrence of systematic error associated with the predominant publication of positive results of studies (results describing statistically significant group differences).
To qualitatively assess the presence of such systematic error in the meta-analysis, one usually resorts to constructing a funnel-shaped scatter diagram of the results of individual studies in coordinates (effect size, sample size). When studies are fully identified, this diagram should be symmetrical. At the same time, there are also formal methods of assessing the existing asymmetry.
Figure 2. Forest plot presenting results of meta-analysis
The results of a meta-analysis are usually presented graphically using a “forest plot” (point and interval estimates of the effect sizes of each of the studies included in the meta-analysis) and in the form of tables with the corresponding statistics.
Systematic review and meta-analysis from Digital Health Outcomes
The Digital Health Outcomes team has extensive experience conducting meta-analyses and systematic reviews using state-of-the-art software tools. Our research is guided by the recommendations of the Cochrane Handbook on Systematic Reviews of Interventions and the methodological requirements of the National Institutes of Health.